Market Disruption with Real Time Data Insights
- michaelthould
- Apr 26, 2018
- 3 min read
Updated: May 30, 2019
Technology is changing our world at a rapid rate. For companies to stay competitive they must adapt to the changing market conditions. This is particularly true for Data Analytics which is now moving into, what many call, the third wave – Real Time Data Insights.
Initially the storing and processing of data was compartmentalised either by department or by different product groups. Operational data was also used to provide data insights with a departmental view.
Customer orientation changed this in the 1990ies, with the need to understand and serve customers better, organisations started to build their analytics models around customer segments. This was supported by the rise of Data Warehouse systems that allowed a centralised view of the data across departments and products. Operational data was replicated, cleansed and enhanced to allow different views on customer segments and other relevant business views. The problems arising from this model was a costly transformation process coupled with the difficulty to integrate insights into operational processes and systems.
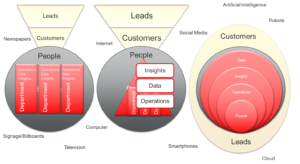
In recent years with the ubiquity of the smartphone revolution customers have become inherently more closely connected to organisations and expect personalised service for a more meaningful customer experience. This puts new challenges on organisations to identify the customer, rapidly classify them based on their behavioural patterns and turn these insights into a better, more personalised service or buying experiences.
The Third Wave
The other remarkable trend is that cloud computing provides a more cost-effective model to tailor analytics platforms utilising vast amounts of computing power and storage at reasonable prices. This allows companies to integrate analytics outputs in real time with operational systems. This improves and automates many customer interactions and provides better information to business staff.
This model also allows organisations to integrate machine learning and AI capabilities. Whilst not entirely new concepts, cloud computing has enabled organisations to use intelligent processes that automate the interaction with customers as well as improve the analytics capabilities.
Foundation to Success – Real Time Analytics Architecture
When building a new capability, the first step is to lay the foundation in form of an architecture that will not only allow traditional batch analytics, but also provide a speed layer that can quickly transform millions of transactions into insights that can help frontline staff better serve the customer and personalise the customer experience.
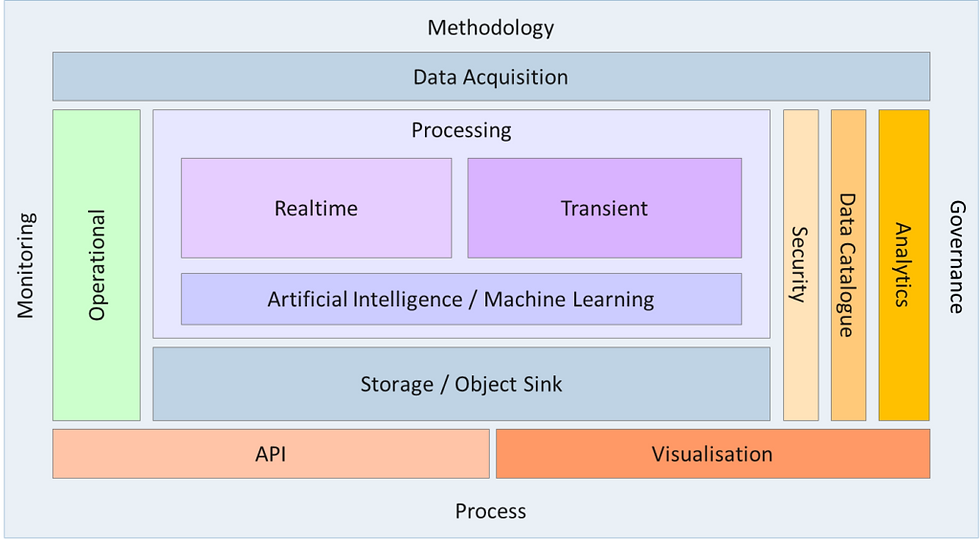
Figure 1: Data Lake Context Diagram
At the core of the modern analytics platform sits an auto scalable processing capability in the form of clustered compute power. The most commonly used technology is Hadoop based clusters running a variety of different applications such as; Spark, Storm or Presto. The clusters can run in long running instances or as transient clusters that only live as long as the analytics process is running and are shut down immediately afterwards.
Modern cloud concepts such as infrastructure as code make it possible to provide flexible Continuous Integration(CI)/Continuous Deployment(CD) processes that stand up a new environment of hardware and software automatically within minutes. Other operational concepts allow Dev Ops teams to manage the data pipelines including reconciliation capability that is paramount when running integrated workloads.
The second important cornerstone of a successful analytics architecture is cheap blob storage with the ability to store large amounts of raw data at low cost. This storage is used as a staging area as well as for long term storage of raw data.
New data access and ETL tools allow direct analysis of the raw data. This enables Data Scientists to find new data patterns directly in the raw data. Working with raw data is a major improvement to the Extract, Transform and Load(ETL) processes in traditional Data Warehouses as it allows for unknown patterns to be retained in the data that would otherwise be lost.
To utilise the full potential of Realtime Data Analytics event driven access is required. This allows downstream systems to be notified when business insights and/or business events (represented as changes in data) are occurring that require timely responses. The service layer also requires an access model that can be provided either in form of APIs or other data access implementations.
Realtime Analytics will increasingly be an integral part of modern Enterprise Data Models enabling organisations to provide personalised services that will disrupt traditional market models. Progressive organisations should move into this space quickly with some low risk projects to understand the potential and complexities of modern analytics platforms. Disrupt or be disrupted!
Let Fusion Professionals work with you to help move your organisation towards a “Real Time” data insights landscape.
Comments